Machine Learning-Derived Intraoperative Warning Systems
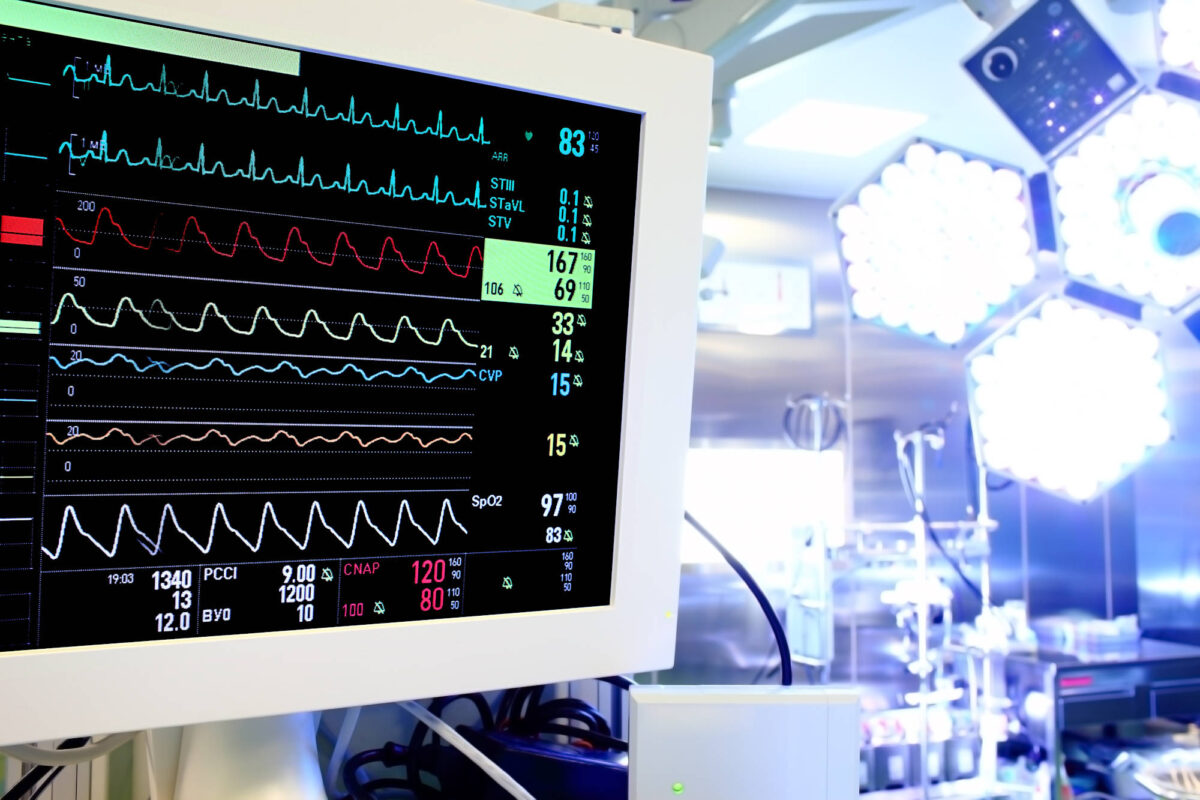
Intraoperative hypotension occurs when a patient’s Mean Arterial Pressure (MAP) drops below 65 mmHg during surgery—the lowest tolerable average blood pressure during an operation [1]. In non-cardiac surgery, this is associated with several postoperative complications, such as acute kidney injury (AKI) and myocardial infarction (MI), and is therefore correlated with higher rates of postoperative mortality [2] [3] [4]. As such, it is imperative that practitioners predict and proactively treat this condition. Intraoperative warning systems could support surgical teams. Current practices, however, are reactive, and involve continuous blood pressure monitoring during surgery. A randomized trial has shown that this significantly lowers the rate of intraoperative hypotension when compared to intermittent monitoring [5].
However, Hatib and colleagues have developed an algorithm—the Hypotension Prediction Index (HYPE)—to predict intraoperative hypotension about 15 minutes before it occurs, allowing it to be treated proactively [6]. HYPE was produced via machine learning: by using large data sets of arterial waveforms, Hatib et. al.’s computer program learned to detect subtle changes in the waveforms—undetectable by practitioners—that foreshadow hemodynamic instability leading to hypotension. HYPE was trained with an initial data set of 1,334 patient records totaling 545,959 minutes of arterial waveform recording. This was followed by a set of testing data—33,236 minutes from 204 patients—used to externally validate the algorithm by projecting actual outcomes against the computer’s predicted occurrences of hypotension [6]. The algorithm has a sensitivity of 88% and a specificity of 87%. That is, occurrences of hypotension will be correctly predicted by the HYPE 88% of the time, and a lack of hypotension will be correctly predicted 87% of the time. HYPE’s reliability demonstrates the potential of machine learning-based intraoperative warning systems: they can accurately interpret subtleties that would otherwise be overlooked by clinicians.
In a clinical setting, Wijnberge and colleagues compared time-weighted average hypotension between two groups: an intervention group of physicians using HYPE—which sounds an alarm when hypotension is predicted—and a control group [7]. In both cases, traditional continuous blood pressure monitoring was available, allowing the physicians to detect hypotension. The study found significant differences in time-weighted average hypotension (median difference of 0.38 mmHg, P = 0.01), as well as average times of hypotension (median difference of 16.7 minutes, P < 0.01) [7]. Additionally, no adverse events resulting in death occurred in the intervention group, compared to two in the control group [7].
Despite this, the study had several limitations. It used a small, selective sample from a single center. Moreover, the patients in the study were at higher risk of intraoperative hypotension than the average patient [7]. This is important because HYPE is only validated for invasive continuous blood pressure monitoring; its results should not be extrapolated to all non-cardiac surgeries. Though HYPE might have clinical uses for patients at high risk for hypotension, more research is required to determine whether these warning systems will benefit all non-cardiac operations or even rival non-invasive methods. Thus, machine learning-derived intraoperative warning systems function well within the conditions they are developed in, but have yet to demonstrate reliable utility in varied operative settings.
References
1. Salmasi, V., Maheshwari, K., Yang, D., Mascha, E. J., Singh, A., Sessler, D. I., & Kurz, A. (2017). Relationship between intraoperative hypotension, defined by either reduction from baseline or absolute thresholds, and acute kidney and myocardial injury after noncardiac surgery: A retrospective cohort analysis. The Journal of the American Society of Anesthesiologists, 126(1), 47-65. https://doi.org/10.1097/ALN.0000000000001432.
2. Walsh, M., Devereaux, P. J., Garg, A. X., Kurz, A., Turan, A., Rodseth, R. N., … & Sessler, D. I. (2013). Relationship between intraoperative mean arterial pressure and clinical outcomes after noncardiac surgery: Toward an empirical definition of hypotension. Anesthesiology: The Journal of the American Society of Anesthesiologists, 119(3), 507-515. https://doi.org/10.1097/ALN.0b013e3182a10e26.
3. Brady, K., & Hogue, C. W. (2013). Intraoperative Hypotension and Patient Outcome: Does “One Size Fit All?”. Anesthesiology: The Journal of the American Society of Anesthesiologists, 119(3), 495-497. https://doi.org/10.1097/ALN.0b013e3182a10cce.
4. Wesselink, E. M., Kappen, T. H., Torn, H. M., Slooter, A. J. C., & Van Klei, W. A. (2018). Intraoperative hypotension and the risk of postoperative adverse outcomes: a systematic review. British Journal of Anaesthesia, 121(4), 706-721. https://doi.org/10.1016/j.bja.2018.04.036.
5. Maheshwari, K., Khanna, S., Bajracharya, G. R., Makarova, N., Riter, Q., Raza, S., … & Sessler, D. I. (2018). A randomized trial of continuous noninvasive blood pressure monitoring during noncardiac surgery. Anesthesia and analgesia, 127(2), 424. https://doi.org/10.1213/ANE.0000000000003482.
6. Hatib, F., Jian, Z., Buddi, S., Lee, C., Settels, J., Sibert, K., … & Cannesson, M. (2018). Machine-learning algorithm to predict hypotension based on high-fidelity arterial pressure waveform analysis. Anesthesiology: The Journal of the American Society of Anesthesiologists, 129(4), 663-674. https://doi.org/10.1097/ALN.0000000000002300.
7. Wijnberge, M., Geerts, B. F., Hol, L., Lemmers, N., Mulder, M. P., Berge, P., … & Veelo, D. P. (2020). Effect of a machine learning–derived early warning system for intraoperative hypotension vs standard care on depth and duration of intraoperative hypotension during elective noncardiac surgery: the HYPE randomized clinical trial. JAMA, 323(11), 1052-1060. https://doi.org/10.1001/jama.2020.0592.